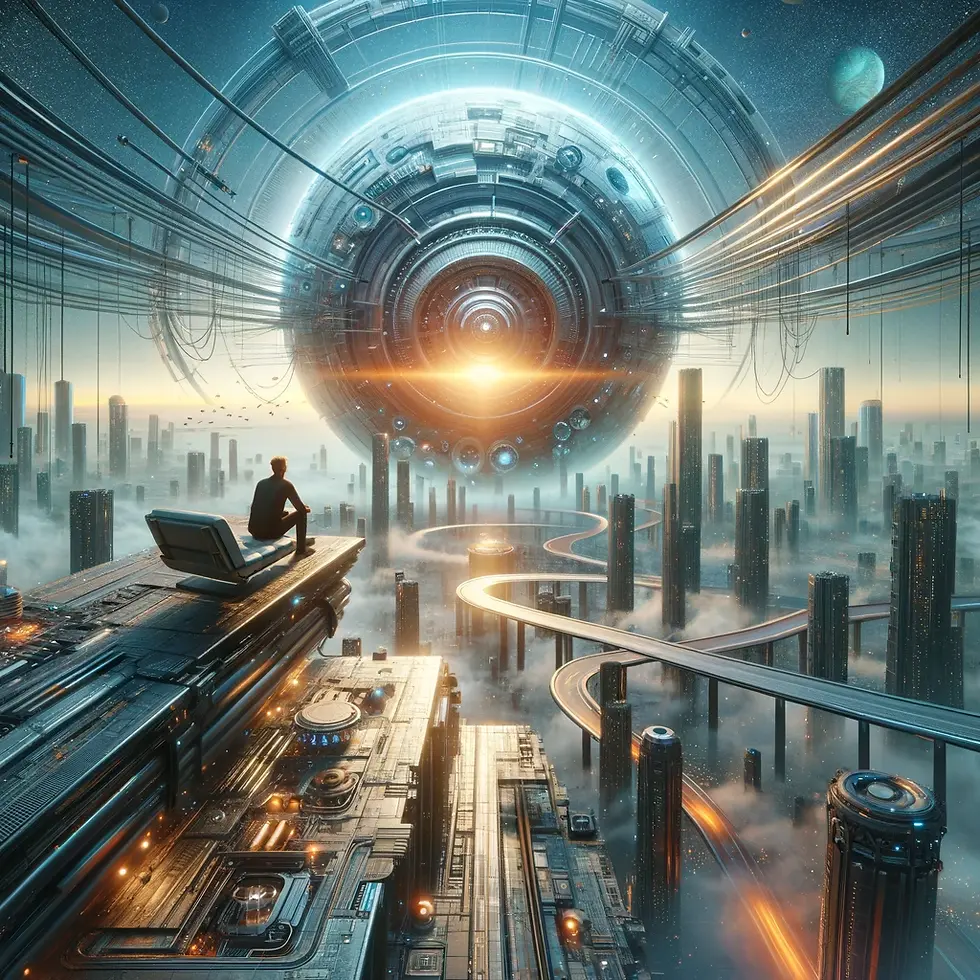
In the expanding universe of big data and cloud computing, the concept of "data gravity" has emerged as a crucial factor influencing where and how organizations manage and process their data. Data gravity, a term coined to describe the tendency for data and applications to attract each other, much like the gravitational force in physics, significantly impacts decision-making processes regarding the deployment of AI workloads.
Understanding Data Gravity
Data gravity refers to the idea that as data accumulates, it becomes more challenging and resource-intensive to move. This phenomenon is particularly noticeable as organizations generate and store vast amounts of data. The larger and more complex the dataset, the stronger its "gravitational pull," attracting applications, services, and other data, thus influencing IT architecture decisions.
For AI engineers, this means that the location of data can significantly affect the efficiency and performance of AI workloads. Large datasets, essential for training sophisticated AI models, can exert considerable data gravity, making it impractical to move them across different environments, such as from on-premises to cloud platforms.
Implications for AI Workload Deployment
The challenge of data gravity is felt acutely in the context of AI workload deployment. AI applications and models often require substantial computational resources and access to large datasets. The decision to run these workloads on the cloud or on-premises is influenced by several factors, including latency, bandwidth constraints, data integrity and security concerns, and cost implications.
Latency and Performance: For AI applications requiring real-time or near-real-time processing, high latency can impede performance. Running workloads closer to where the data resides can reduce latency and enhance application responsiveness.
Cost Considerations: Migrating large volumes of data to the cloud can incur significant costs, including data transfer and storage expenses. An on-premises deployment might be more cost-effective for AI projects with substantial continuous computational needs.
Security and Compliance: On-premises infrastructure offers greater control over data, which is crucial for compliance with regulatory standards and protecting sensitive information.
Strategies for AI Engineers
AI engineers must navigate the complexities of data gravity when deciding where to run their AI workloads. Here are some strategies to consider:
Hybrid Solutions: Combining the benefits of both cloud and on-premises infrastructure can offer a balanced approach. Sensitive or heavily used data can remain on-premises, while less critical workloads leverage cloud scalability.
Data Tiering and Management: Implement data tiering strategies to classify data based on access frequency and importance. This can reduce the volume of data that needs to be actively moved and managed, alleviating the impact of data gravity.
Edge Computing: For applications requiring immediate data processing, edge computing can process data closer to its source, minimizing the need to transfer large datasets to centralized data centers or clouds.
Incremental Migration: Consider migrating data in smaller segments, prioritizing essential data and workloads. This approach can help manage bandwidth and minimize disruptions.
Conclusion
Data gravity is a significant consideration for AI engineers planning the deployment of AI workloads. By understanding the implications of data gravity and employing strategic approaches to manage its effects, AI professionals can optimize the performance and cost-effectiveness of their AI applications. The choice between cloud, on-premises, and hybrid solutions should be informed by a thorough analysis of data management needs, performance requirements, and long-term strategic objectives.
Comentários